Economic Forecasting for Thailand using Predictors with Different Frequency
DOI:
https://doi.org/10.14456/mjba.2020.1คำสำคัญ:
Elastic Net Regression JEL Classification Codes: C36, C53, C55, E17, GDP, Growth, MIDASบทคัดย่อ
This study examines economic growth of Thailand using predictor variables with different frequencies (yearly, quarterly, and monthly). Mixed Data Sampling (MIDAS) is approached to combine the enormously different frequency data. Ridge, LASSO, and elastic net regression are also used to specify factors affecting to Thailand economic growth. Data have been carefully collected from January 2000 to December 2019, total 20 years. The empirical results show that variables with positive impact on GDP growth consist of industry value added (INDUSVA), tax revenue (TAXREVEN), electricity consumption (ELECC), and investment growth (INVEST), while negative impact of external debt (EXD) on growth also exists.
References
Abeysinghe, T., & Rajaguru, G. (2004). Quarterly real GDP estimates for China and ASEAN4 with a forecast evaluation. Journal of Forecasting, 23(6), 431-447.
Acemoglu, D. (2009). The crisis of 2008: structural lessons for and from economics. Globalization and Growth, 37.
Acemoglu, D., Johnson, S., & Robinson, J. A. (2002). Reversal of fortune: Geography and institutions in the making of the modern world income distribution. The Quarterly journal of economics, 117(4), 1231-1294.
Ali, S., Alam, K. J., & Islam, M. S. (2016). Effects of trade openness and industrial value added on economic growth in Bangladesh. International Journal of Sustainable Development Research, 2(2), 6.
Andreou, E., Ghysels, E., & Kourtellos, A. (2010). Regression models with mixed sampling frequencies. Journal of Econometrics, 158(2), 246-261.
Barua, A., & Chakraborty, D. (2006, July).
Barua, A., & Chakraborty, D. (2006, July). Liberalization, trade and industrial performance: an empirical analysis for India. In paper for the 2nd APEA Conference, held at the Center for International Economics, University of Washington, Seattle.
Clements, M. P., & Galvão, A. B. (2008). Macroeconomic forecasting with mixed-frequency data: Forecasting output growth in the United States. Journal of Business & Economic Statistics, 26(4), 546-554.
Cooray, A. (2009). Government expenditure, governance and economic growth. Comparative Economic Studies, 51(3), 401-418.
Durlauf, S. N., Kourtellos, A., & Minkin, A. (2001). The local Solow growth model. European Economic Review, 45(4-6), 928-940.
Ferrara, L., & Marsilli, C. (2013). Financial variables as leading indicators of GDP growth: Evidence from a MIDAS approach during the Great Recession. Applied Economics Letters, 20(3), 233-237.
Ghysels, E., Sinko, A., & Valkanov, R. (2007). MIDAS regressions: Further results and new directions. Econometric Reviews, 26(1), 53-90.
Ghysels, G., & Valkanov, R. (2006). Linear time series processes with mixed data sampling and MIDAS regression models. mimeo.
Grabova, P. (2014). Corruption impact on Economic Growth: An empirical analysis. Journal of Economic Development, Management, IT, Finance, and Marketing, 6(2), 57.
Hicks, J. (1969). A theory of economic history (Vol. 9). Oxford: Oxford University Press.
Li, J., & Chen, W. (2014). Forecasting macroeconomic time series: LASSO-based approaches and their forecast combinations with dynamic factor models. International Journal of Forecasting, 30(4), 996-1015.
Marcellino, M., & Schumacher, C. (2010). Factor MIDAS for nowcasting and forecasting with ragged‐edge data: A model comparison for German GDP. Oxford Bulletin of Economics and Statistics, 72(4), 518-550.
Maestas, N., Mullen, K. J., & Powell, D. (2016). The effect of population aging on economic growth, the labor force and productivity (No. w22452). National Bureau of Economic Research.
Rodrik, D. (2000). Institutions for high-quality growth: what they are and how to acquire them. Studies in comparative international development, 35(3), 3-31.
Svensson, J. (2003). Why conditional aid does not work and what can be done about it?. Journal of development economics, 70(2), 381-402.
Thianpaen, N., Liu, J., & Sriboonchitta, S. (2016). Time series forecast using AR-belief approach. Thai Journal of Mathematics, 14(3), 527-541.
The Office for National Statistics. (2019, November 1). Calculation of GDP. Retrieved from https://www.ons.gov.uk/
Tiffin, A. (2016). Seeing in the dark: a machine-learning approach to nowcasting in Lebanon.
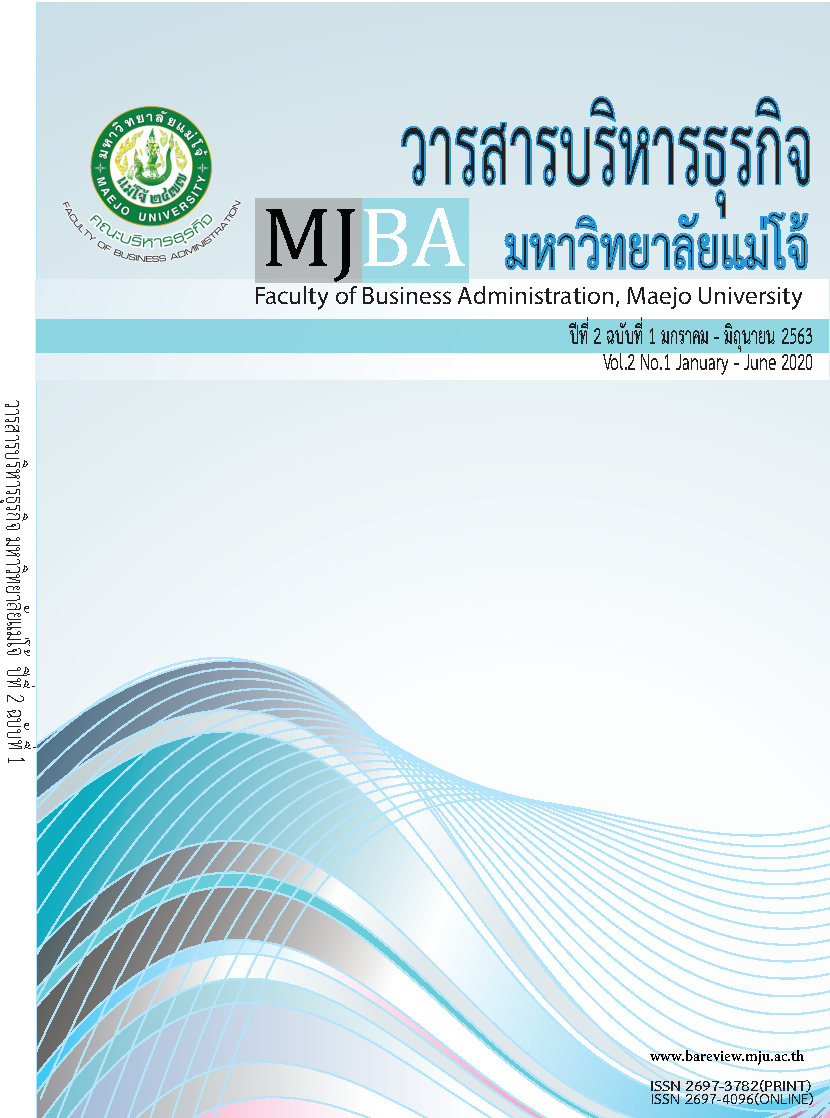